In the modern business landscape, the effective management and utilization of knowledge have become pivotal for staying competitive. As organizations struggle with the sheer volume of information they generate and acquire, the adoption of AI knowledge agents emerges as a compelling solution. These sophisticated tools, powered by advancements such as Retrieval-Augmented Generation (RAG), promise to revolutionize how companies access and leverage their internal knowledge. However, alongside the enthusiasm for these technologies lies a critical consideration: data privacy.
This blog post seeks to explore the intersection of AI knowledge agents and data privacy, focusing on the integration of Retrieval-Augmented Generation. Our goal is to illuminate how businesses can employ this technology to make their knowledge more accessible while ensuring the confidentiality and security of their data remain uncompromised.
The Role of Retrieval-Augmented Generation (RAG)
In the rapidly evolving landscape of artificial intelligence, AI knowledge agents stand out as a transformative force for businesses seeking to navigate the complexities of information management and utilization. At their core, AI knowledge agents are sophisticated AI systems designed to interact with users in a conversational manner, retrieving, interpreting, and delivering information from a vast array of sources.
A pivotal enhancement in the functionality of AI knowledge agents comes from Retrieval-Augmented Generation. RAG represents an approach that leverages the generative capabilities of large language models, such as GPT, and combines them with dynamic data retrieval mechanisms. This integration allows the AI to not only generate responses based on the vast amounts of data it has been trained on but also to pull in the most current, specific information related to the query at hand from various databases, vastly improving the accuracy and precision of the AI-generated content.
The following example underscores the value of leveraging the RAG approach.
A biotech company has generated a large database of documentation about their technology. When the business developer receives inquiries from a potential collaborator, they no longer need to sift through extensive documentation manually. Instead, they can consult the AI knowledge agent, which promptly provides a well-crafted response along with summaries of pertinent documents that informed the AI’s answer. This scenario highlights two critical benefits:
Precise Integration with Enterprise Databases: The RAG framework facilitates the seamless incorporation of the company’s internal databases into the response process. This integration is key to generating accurate and highly relevant responses, a capability that surpasses the limitations of direct interactions with large language models (LLMs) like ChatGPT. By accessing specific enterprise data, the AI can tailor its responses in a way that pure LLMs, relying solely on their pre-trained information, cannot.
Data Privacy Preservation: Importantly, this approach ensures the company’s database remains distinct and separate from the LLM’s knowledge base. The enterprise data does not become part of the LLM’s training material, safeguarding the confidentiality and privacy of sensitive information. This separation addresses a crucial concern for businesses about protecting their proprietary data while still benefiting from the advanced capabilities of AI-driven responses.
This example vividly demonstrates how RAG-based AI agents can transform information retrieval within organizations, making it faster, more accurate, and more secure.
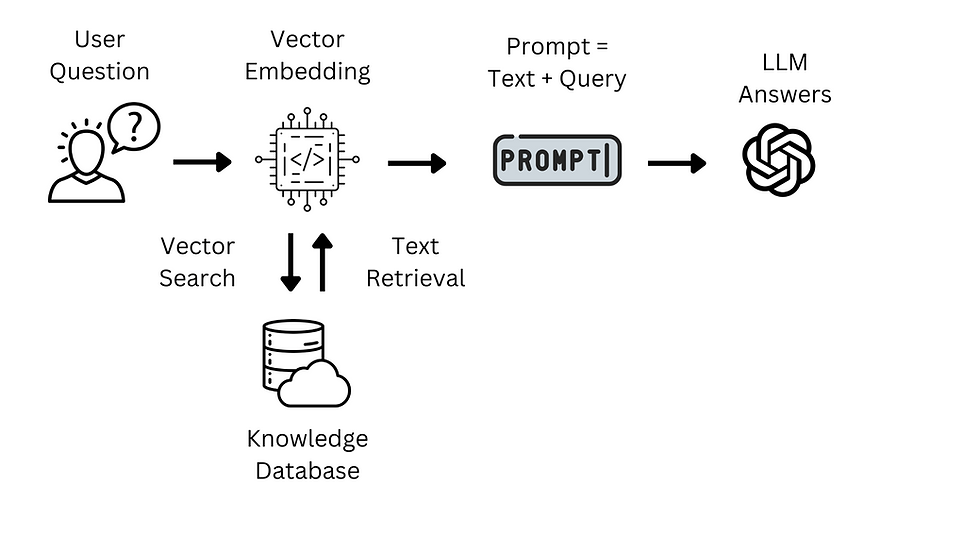
How Does It Work?
RAG employs a sophisticated multi-step process to transform corporate documents into actionable insights. Here’s a breakdown of how it operates:
Building the Vector Database
Data Ingestion: The journey begins with data ingestion, where content from corporate documents (such as PDFs) is prepared for analysis. In this step, the documents are segmented into manageable “chunks” to facilitate further processing.
Embedding: These chunks are then passed through embedding models — neural networks that convert text into numerical vectors representing the text’s semantics. This conversion is crucial for making the text understandable and actionable by the AI models.
Vector Database: All vector embeddings are index and stored in a Vector Database, with each embedding linked to the text it represents, stored in a separate file with a reference to its corresponding embedding. This infrastructure allows for efficient data management and retrieval.
Querying the Vector Database
Embedding Query: When a user’s question is received, it’s converted into a vector using the same embedding model applied to the document chunks.
Retrieve Relevant Vectors: The Vector Database then searches for vectors closely aligned with the user’s query, providing a context-rich foundation for generating responses.
Retrieve Relevant Text: The system fetches segments of documents matching the identified vectors. These chunks of text form the basis for generating an informed response.
Prompt Engineering and LLMs: The retrieved text is then used alongside the original query to create a prompt. This prompt is then processed by an LLM, generating a human-like response based on the input (query + retrieved text) they receive.
This intricate process demonstrates the power of RAG in leveraging corporate knowledge efficiently. By transforming static documents into dynamic, queryable data and utilizing advanced AI to interpret and respond to user queries, businesses can unlock new levels of insight and efficiency.
Conclusion
In an era marked by an exponential increase in data volume, the ability to efficiently process and interpret vast amounts of information swiftly stands as a critical differentiator in the competitive business landscape. AI knowledge agents, particularly those enhanced with Retrieval-Augmented Generation (RAG), represent a significant leap forward, offering businesses the tools to transform this data deluge into actionable insights while ensuring stringent data privacy standards. This integration not only streamlines access to and management of corporate knowledge but also redefines operational efficiency and decision-making processes. As the digital landscape evolves, embracing AI knowledge agents is a strategic imperative, paving the way for a future where data is not just an asset but a catalyst for innovation, safeguarded by robust privacy protections.
For more AI insights follow my Instagram page lorevanoudenhove.ai and LinkedIn page.
Comments